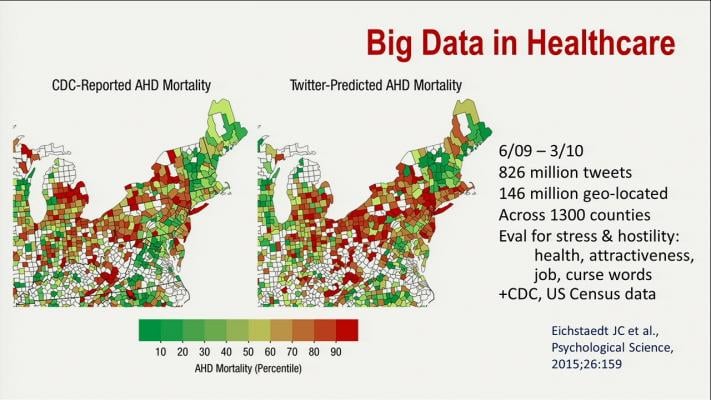
Big data, showing correlation between a CDC study on cardiovascular disease and a study conducted based on hostility in Twitter tweets. This demonstrates how big data from social media might be used to in new ways to evaluate population health.
The buzz term “big data” has made a rapid entry onto the healthcare scene in the past couple years with promises of improving healthcare, but there are still many who are trying to figure out how exactly it will accomplish this. Efforts were made to explain big data and its application to healthcare at the American College of Cardiology (ACC) and Healthcare Information and Management Systems Society (HIMSS) meetings earlier this year.
This perception is mainly due to the current state of health IT, which leaves a lot to be desired due to problems with interoperability and bottlenecks to sharing data from disparate software systems. However, as enterprise imaging and information systems become the new normal in healthcare, enabling the free flow of structured data across healthcare systems and health information exchanges, big data will play a major role. At the department level, analytical software can now identify workflow or standard-of-care issues so they can be corrected or made more efficient. This includes identifying and quantifying workflow improvements of new versus old equipment to justify return on investment (ROI) for capital replacement costs (i.e.: CT, CR, angiography systems, MRI, etc.). The analytics also can monitor data and set alerts for everything from radiation dose and patient throughput at clinics on specific machines using specific exam protocols, to procedural times in the cath lab based on each lab, physician and patient type.
These sorts of comparisons will become very important in the coming years for comparisons in care and how one center or department does something compared to others in the health system to improve ROI, efficiency and outcomes. As Medicare moves from fee-for-service to a value-based and bundled payment system, this data can be used to figure out the best strategy to diagnose or treat specific types of patients to reduce costs.
“We are falling short of achieving meaningful use of health information technology,” said James Tcheng, M.D., FACC, FSCAI, Duke University, North Carolina, during a presentation on big data at ACC 2015. “We are focused on administrative click-off boxes, but data is now being used to help identify which patients we need to engage, and this is a paradigm shift. I think big data will become a big part of what cardiovascular care will look like in the future,” he explained. “Big data is really a vision of an interoperable health data infrastructure, and it will be a big driver in the future for research and how we provide care in the future,”
Data Mining Electronic Healthcare Data
With the conversion to electronic medical records (EMR), Tcheng said the amount of data produced is vast and contains information that can now be easily accessed with electronic data mining, which was impossible with paper records. He said a typical tertiary care hospital generates about 100 terabytes (TB) of data per year. By comparison, he said the Library of Congress is estimated to contain only about 10 TB of text data.
“Your healthcare institutions each generate more data per month than the entire Library of Congress," he said.
Tcheng said the Library of Congress audio and video file collections add an additional 20 pedibytes (PB) of data to the overall count. By comparison, he said Google uses about 24 PB of data per day and the Duke Heart Center where he works processes about 30 terabytes of clinical data per year, including reports, images and waveforms.
This volume of data offers new sources of healthcare insights that previously were very difficult and time-consuming to manually tabulate. Today, electronic data mining allows fast-access data points that are currently buried in mountains of documents. Take, for example, the ability to find heart failure (HF) population trends based on region, state, county or individual hospital. These factors might include pulling vital signs trends from yearly exams or family history from EMRs to predict which patients should be screened for HF years before they present with HF symptoms. Analytics showing low-income areas with a high incidence of HF acute care episodes in emergency departments might help target prevention outreach programs or help earmark federal funds. This large amount of data might also help identify new ways to curb HF readmission rates or better engage patients to manage their condition.
Tcheng said one roadblock to processing existing data is that 95 percent of it in the world today is unstructured. This leads to issues when different doctors or institutions use different terminology for the same thing. This variability makes meaningful data mining very difficult. This is why structured reporting with standardized taxonomy and definitions is so critical moving into the future.
“Unfortunately, most healthcare data is also unstructured, so how do we sort all that data?” Tcheng said. “It’s not about what the doctor collects in the office anymore, it’s about how we take all this data from various sources and aggregate it.”
New Sources of Healthcare Data and Research Models for Population Health
Tcheng said big data from outside healthcare may offer new avenues of research. He pointed to a study[1] that used mass data mining from Twitter tweets in the northeastern United States. The study looked at measures for stress based on the use of hostile language, profanity and the expression of negative feelings using key words or terms. This was used to create a color-coded map by county to predict the incidence of heart disease. Tcheng showed the resulting map side by side with a similar map created by the Centers for Disease Control (CDC) based on tedious clinical data analysis from hospitals. The two maps nearly matched and showed a compelling example of how mainstream big data sources like Google, Facebook and other popular electronic media can be leveraged for serious healthcare research.
“Social media might be a better predictor than all the studies we do to better identify areas where more care is needed for cardiovascular disease,” Tcheng explained.
Mining Electronic Medical Records and Patient Reports for Population Health
A subset of healthcare big data is “population health,” where data from a hospital or the whole healthcare system is mined for numerous data points to determine patients who may need additional care. This can enable a hospital to boost revenue with additional services to its existing patient bases, while helping to reduce healthcare costs downstream by preventing care acute episodes. This may include reviewing all cardiovascular patient data to determine which patients have symptoms of peripheral artery disease to bring them in for a screening that will likely lead to additional lab, exam and procedural workups that are needed. Another example might be mining records to determine all current patients who may qualify for transcatheter aortic valve replacement (TAVR) procedures under U.S. Food and Drug Administration (FDA) guidelines if the hospital is considering building a TAVR program or looking to fill an existing program with patients. Population health will also help identify patients who are most likely to develop chronic conditions based on available data, such as heart failure, diabetes or chronic obstructive pulmonary disease (COPD). Being able to predict which patients should be screened pre-emptively to begin early interventions and lifestyle changes could have a massive impact on the healthcare system. These three diseases alone are among the most costly to treat and manage after a patient reaches the later stages, which is generally when they seek medical help and are the cause of regular re-admissions.
On a higher level, population health data for thousands of patients can be monitored by software to identify outbreaks of disease, or disease hot spots. This includes identifying outbreaks of flu or other epidemics in real time, or cancer hot spots of patients that all live or work in the same location.
Big data will help identify patients who fall through the cracks currently and help clinicians follow up with them, such as those who have not had a doctor's visit in years, those with disease symptoms who never came back for treatment or follow-up, or those who were treated for an acute condition (heart attack) but the patient has not seen a doctor in 2-3 years since and require follow-up care to prevent another heart attack. These types of things seem small, but will go a long way to preventing patients from showing up in the emergency room, which reduces overall healthcare costs in the long run. This also helps boost income for health systems to keep patients coming back for checkups.
Watch the video, "Population Health to Identify High Risk Cardiovascular Patients."
Big Data Aids Decision Support For the Best Outcomes
Clinical decision support (CDS) software can help decide appropriate medications, detect potential interactions, and determine the most appropriate tests, imaging exams and procedures for patients with specific symptoms or disease states. Doctors have long argued CDS might take away their decision-making power to manage patients. However, as healthcare continues the trend of following evidence-based medicine, some physicians still do not follow appropriate use criteria (AUC) set by their own specialty societies, leading to poorer patient outcomes. From this perspective, CDS looks like a more attractive option to hospital administrators from a liability and reimbursement standpoint. This is being further reinforced by Stage 3 Meaningful Use requirements for electronic medical records (EMR) and the latest amendments to Medicare, which now require CDS records to support reimbursement claims by 2018. In the future, CDS justification documentation might be required for full Medicare reimbursement.
The major issue with creating a single software source for CDS information is that guidelines constantly change and there is a constant flow of new clinical data that can rapidly change the standard of care.
“We know when you comply with medical guidelines in cardiology you have better outcomes,” Tcheng said. However, he said one obstacle to CDS use has been software able to keep track of all the new information in real time.
He said big data search engines will increasingly be used by software vendors to aggregate data from the latest clinical trials, studies and society AUC guidelines for various societies into applications that monitor orders physicians enter electronically. These types of CDS software will flag any orders than do not meet AUC and help clinicians make more appropriate decisions on everything from which medications to which imaging tests are best suited for a specific patient. It is hoped following AUC based on constantly updated patient outcomes data will help reduce the number of tests ordered and better guide therapy choices known to offer the best outcomes. Medicare expects this will help reduce healthcare costs in the future, both by reducing the number of tests performed and by picking treatments that have the best measure of success.